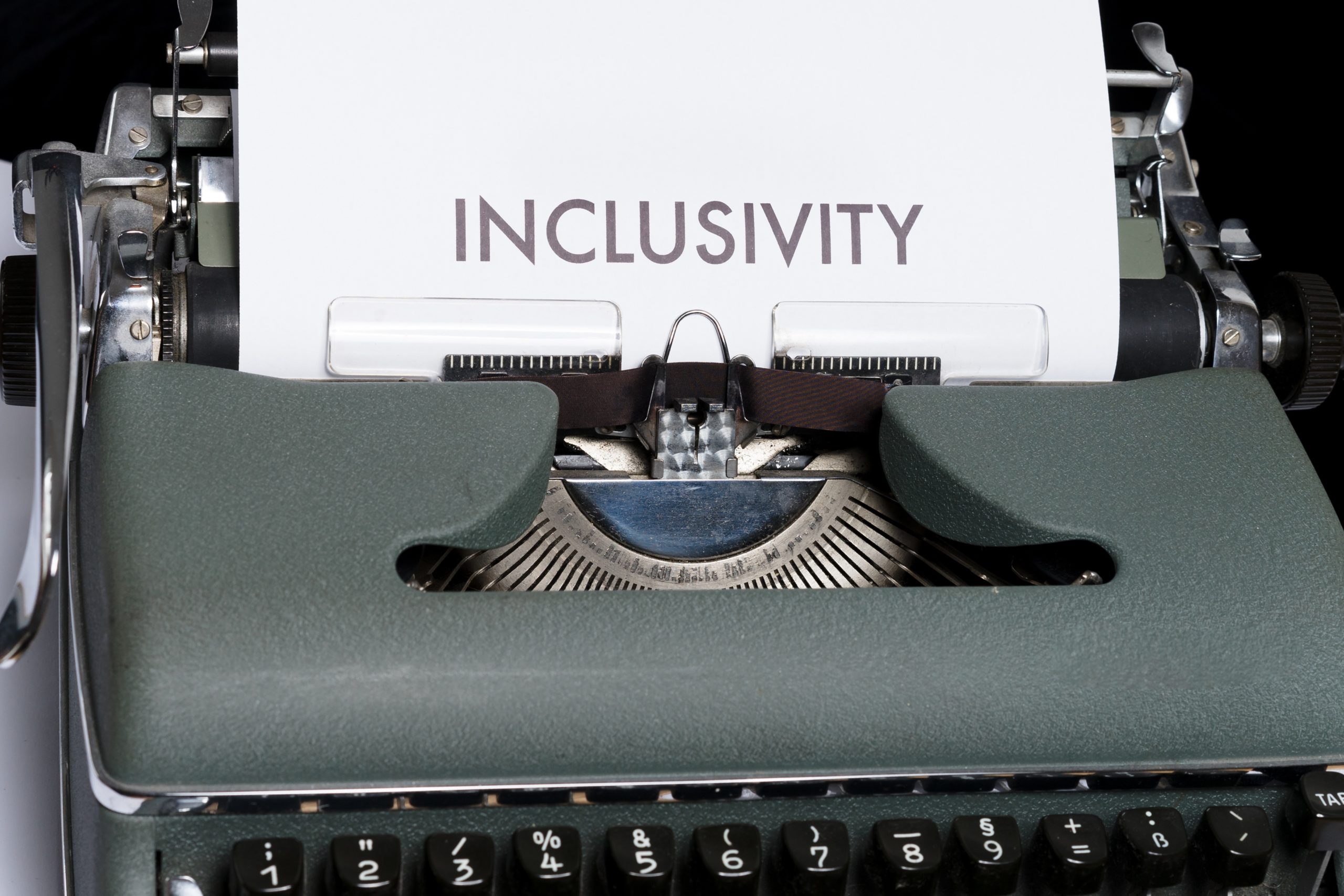
On Racial Justice and Algorithmic Bias
Over the last few weeks, social justice topics have exploded across all media in light of recent unfortunate events in the United States. Hand-in-hand, the AI community has also seen a tremendous amount of conversation across multiple social media platforms on algorithmic bias and its implications. While social bias and AI bias are closely interlinked, racial bias has created a flywheel effect to increase the visibility of algorithmic bias. If you missed the Twitter back-and-forth between two leading AI Bias Experts, Timnit Gebru and Yann LeCun, this article gives you a fairly comprehensive review of the drama. Data bias is a critical issue, but it represents only one aspect of bias that can enter an AI solution.
I went down the rabbit hole to understand the current challenges in facial recognition and read/listened/watched some of the most prominent Black Women in AI voices – Joy Buolamwini (Algorithmic Justice League), Deb Raji, Timnit Gebru, Renee Cummings, and Ruha Benjamin. Some of the questions being raised are:
- How can AI be applied to minimize existing social bias rather than reinforce it?
- While exploring AI for Good, why are we not looking at AI doing Bad?
- How significantly can we minimize AI bias problems with diverse representation across the ML lifecycle?
I was really impressed with the GenderShades project that investigated gender classification error rates with commercially available facial recognition products and the follow-up publications with Actionable Auditing and Saving Faces. I strongly recommend Deb Raji’s recent podcast, How External Auditing is Changing the Facial Recognition Landscape, for anyone new to this domain to ramp up quickly on critical issues that still continue to fester. We began with Model Cards for Model Reporting and Datasheets for Datasets but have a long way to go for creating accountable AI. If you really enjoyed the podcast, check out the complete TWIMLAI Bias in ML playlist.
The AI For Good Global Summit recently held a special web session for the Gender Breakthrough Track. The session was specifically focused on a call for submissions towards AI Technologies to Achieve Gender Equity to prevent the cycle of algorithmic bias. Three topics were presented towards a competition for proposals (due end of July)
- Identify technical and non-technical ways to define, detect and evaluate algorithmic gender bias
- How can AI systems be designed and used to help human decision making be more gender inclusive?
- How can diverse data sets be identified and collectively leveraged to give a more complete picture on gender inequality to allow for evidence-based policy making
Will you apply?
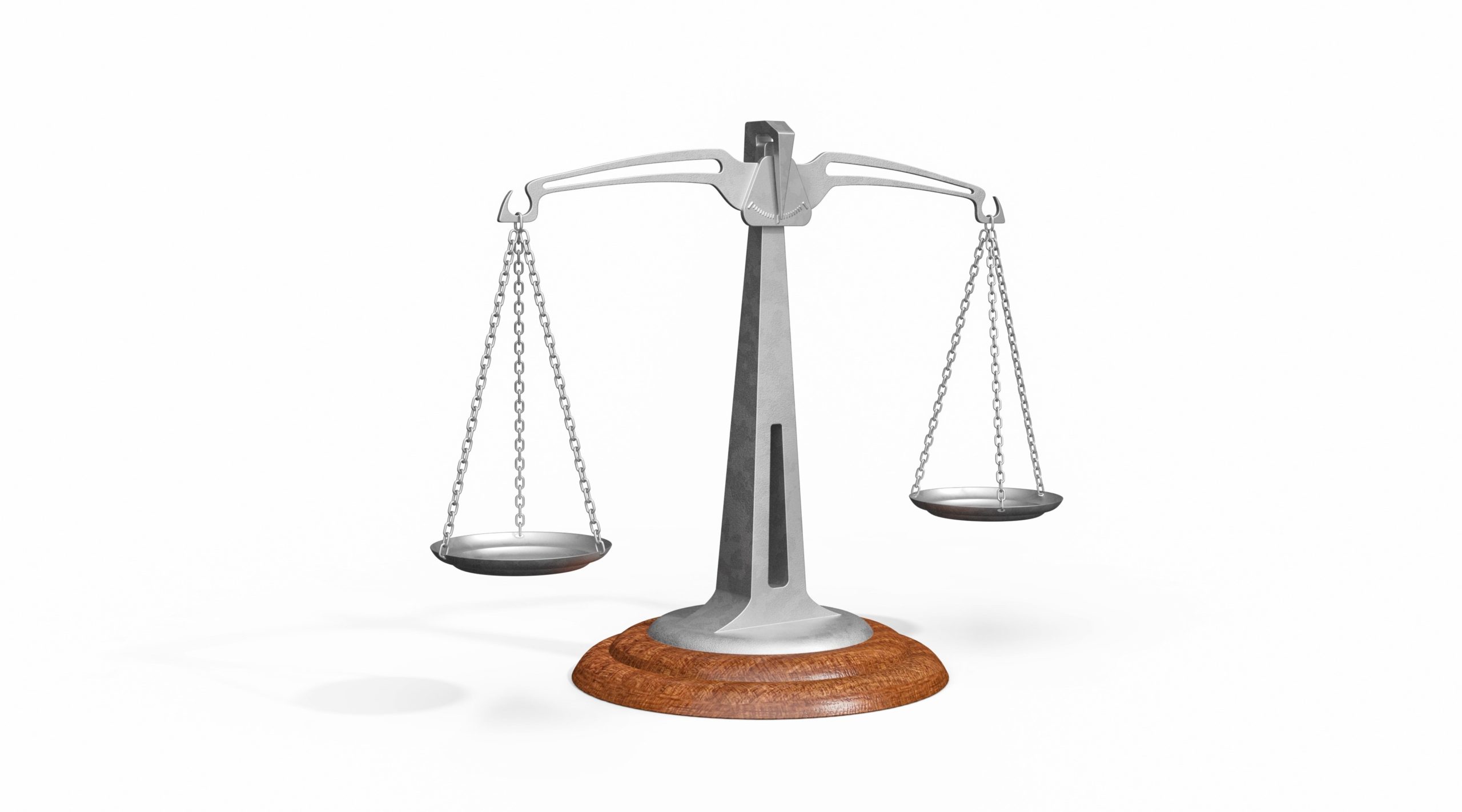
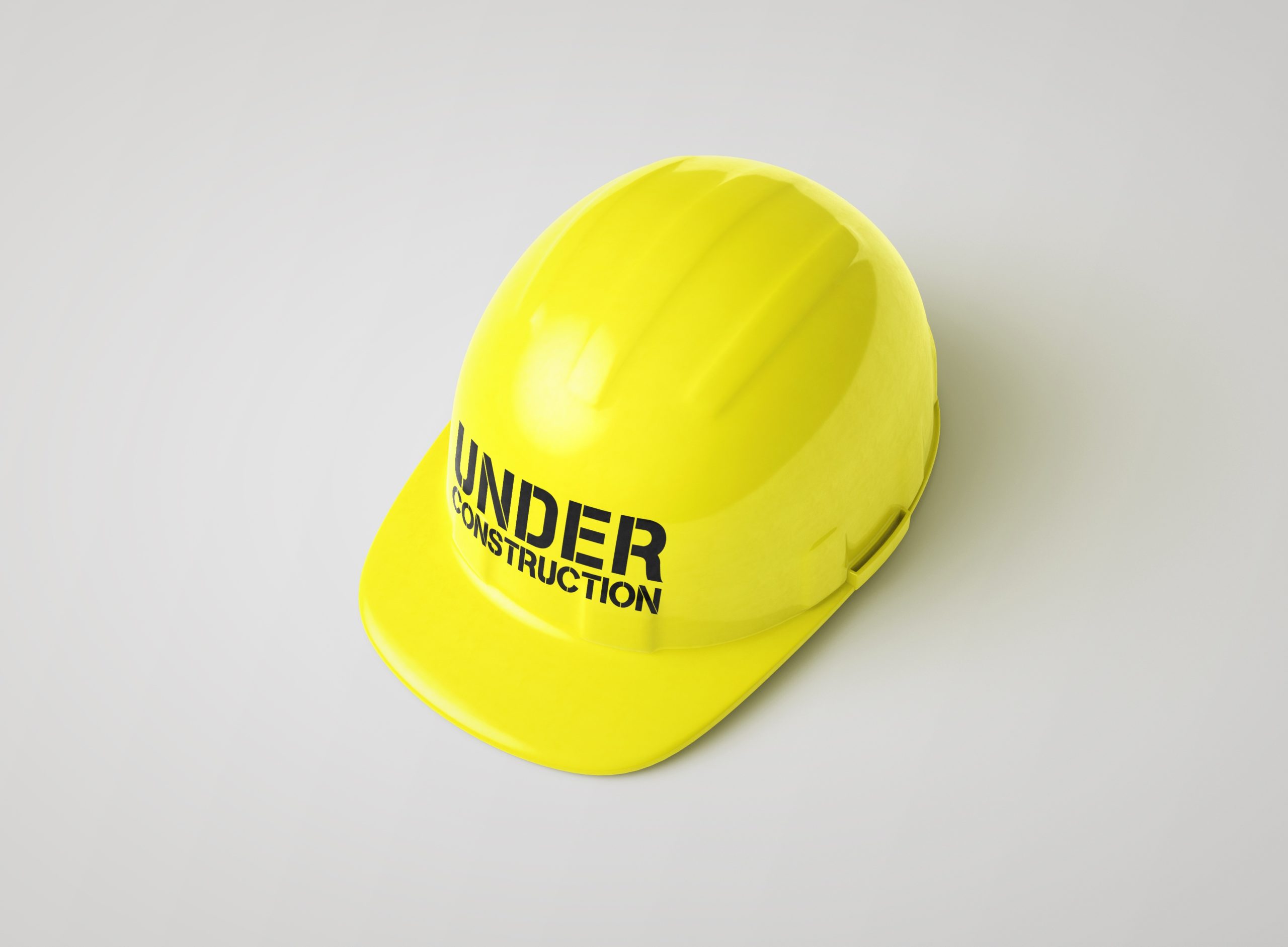